SPEAKERS:
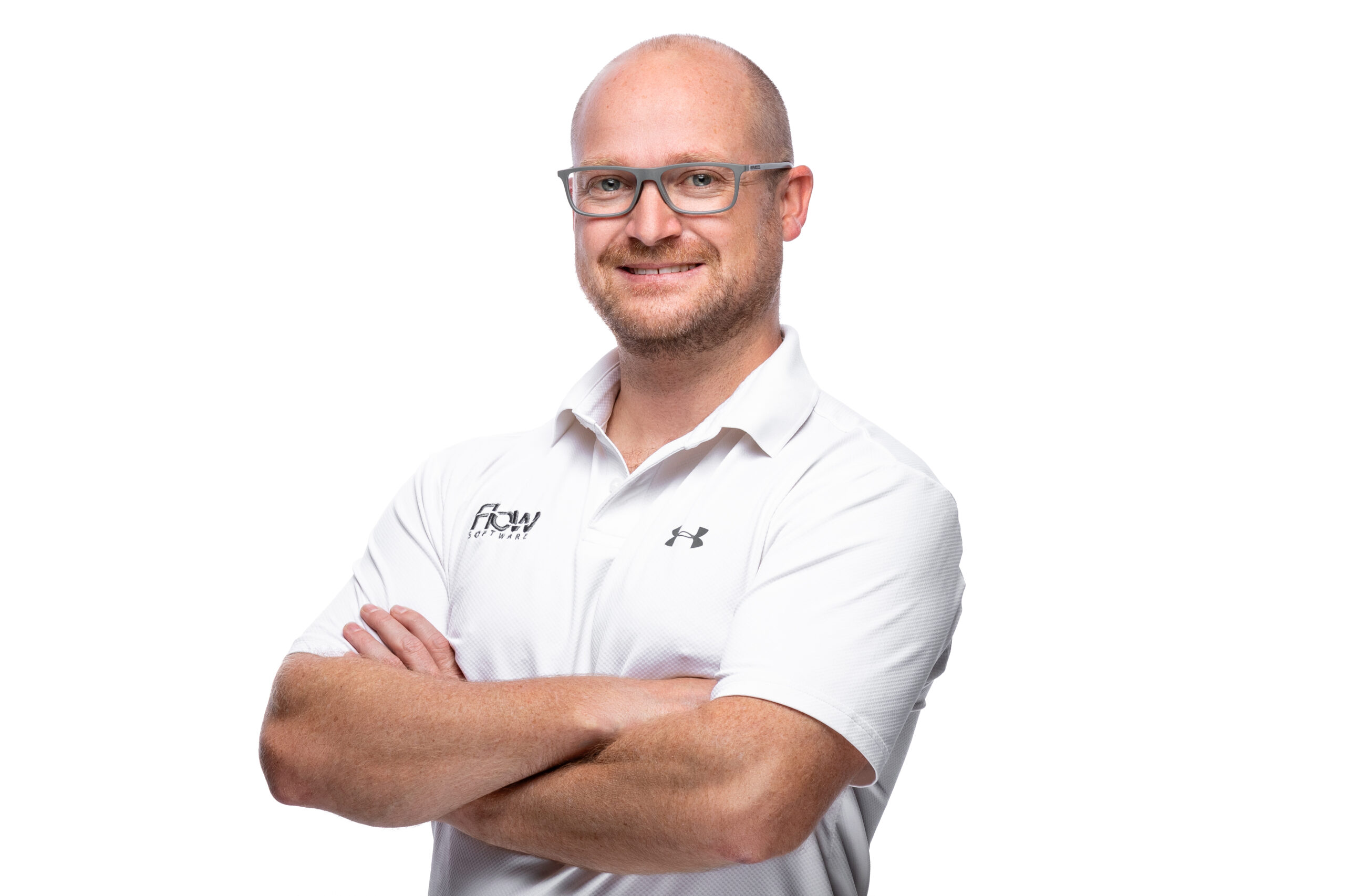
Introduction
While we recognise that our community includes many specialists, the fundamental sessions served as an introduction to Flow for many new members in attendance – the features and benefits and why and how you would deploy Flow. The recording serves as a fantastic recap for those with limited experience!
Transcript
00:01
All right, thanks, Clark. Thanks, Kent. It is separate. It’s not Clark Kent. My name is Lenny, and I am what stands between you guys and lunch. So just bear a bit with me. I do have some funky socks as well. I think our funky socks are the best. They’re really cool. So there’s some questions, and I want some participation, please. All right, so my name is Lenny. I’m the customer success manager at Flow Software. And if you haven’t realized it by now, we started with real time data. We moved that data into the canary historian, and now we’re going to do some analytics on top of that data with flow. Cool. So let’s kick it off with a few questions here. What do you guys think is key for analytics? A, b, or c? There’s no d. All of the above.
00:57
So it is not a trick question. What do you guys think? Socks is on the line. Let’s give somebody else a chance. You already got some socks. You got a water bottle? Okay, cool. Oh, okay. That’s fine. Off we go. Shoot with answer. All of the above. No, sorry, wrong. I’m going to give you guys a guess. It’s what Clark talked about just now. Yes, historical data. There we go, sir. Oops, sorry. That’s horrible. I might predict the rugby score, but I can’t pass. All right, so definitely we need some historical records to do analytics on. All right, so let’s put some values on the display. There’s two values. What can you guys tell me about those two values? One is high and one is low. I don’t know. Can’t read it. It’s too small. Okay.
01:52
The one says 87, and the other one says 36. What does it mean? Nothing. All right, we need some context to these data points. All right, so let’s add some context. Now, the first piece of context that we’re going to get is the real time context that we’re going to get where we’re actually collecting the data from. We’re going to get a model associated to that point. There’s a very nice. I don’t know how this laser works, but there’s a nice model associated with it. A nice ISO 95 breakdown or structure to that point. Other kind of context that a point at the edge can gives us as unit of measures, it can gives us ranges. So all of that is context that I can add to the edge. All right? So at least I know, okay, this is a process variable.
02:39
I can see it’s a pv tag, and I know exactly where this is located. Within my facility. So the edge can give me that kind of context as well. What other context can we add to these data points? Well, the second piece of context that we can get is the historical data context. All right, so let’s look at this as a trend. Aha. That gives you some kind of indication of what’s happening, right? So now at least I know it’s a totalizer value. It’s a totalizer value that tells me how much energy or flow or whatever process variable that is that I’m actually using on the plant floor. How many KPIs do you guys think can we generate of only those two points? Rough guess.
03:28
How many KPIs do you think that these two points can generate out of that data on your historian turning it into information, say again? Five. At least five. Good. It’s a good number. We’re going to end up with around 19. Okay, so let’s bear with me. All right, so here we go. We’ve got power usage, that is the blue data point, and we’ve got our total production, which is the white data point. Powered power usage is a raw pv. Right. And you’ll notice that there’s a little bit of anomaly on that data point there. All right, now what do we want to do? Do we want to use that data point when we create our KPIs or calculations? Potentially not. It depends on what the rule is or what the use case is.
04:19
If I’m going to use that data point in a min or a max aggregate, well, that’s going to skew the number a little bit. Right. So the very important first thing that we need to do with our data is we need to cleanse it. We need to put in some rules, some data cleansing rules to make sure that this data is scrubbed and that we can actually use this data within our analytics. So within the flow engine, what we can do is we can build in these rules. We can take the raw tag from the historian. We can say, you know what, eliminate bands, eliminate stale values, and potentially replace those values with either a check weigher or a default value, whatever the case.
04:54
In this case, I’m just going to remove it from the data set completely so I can create a rule that will go and eliminate any bad data to my KPIs that I want to go and generate. All right? So that’s the first thing that we’re going to do is we’re going to scrub it. We’re going to make sure that data is right and ready for data aggregation. Let’s create some context. Now, the first thing that we’re going to do with context is we’re going to create context based on time intervals or time buckets. So you’ll notice these little bands here that represents hourly buckets. And I’m going to go and create hourly KPIs based on that. So what that’s going to give me is my hourly energy usage. It will also give me my hourly production. All right?
05:36
And we have different types of aggregation methods that we actually can go and apply to these buckets to create that. But let’s look at practical use cases. So you’ll notice that there’s some points in my production where I’m not actually producing any product, all right? That’s when either I’m cleaning the line or I’m busy with a setup for a new process to actually happen on that plant floor. So I would like to know, you know what, while the line is idling or standing still, is it actually using a lot of power? That’s a nice KPI that I can use. So I can use these sections in the process to then tell me what is my energy usage per cleaning cycle. And very importantly, I’m using the signal from my production value in conjunction with the value from the actual energy meter.
06:33
So I’m being able to mix and match data points to create these calculations and aggregations. All right, but what about other data? We talked about data silos and where other data can come from. And definitely our mea systems that run on our plants can provide additional context as well. Now I would like to know, you know what, that’s great, but I would also like to know what was my total production per batch. Right? So I’m going to get data from my MEas system. I’m going to overlay the production events with the data coming from the historian, right? And I can do that.
07:09
And I can create additional KPIs based on that data points where I can now have what is my total energy used per batch or what is my total energy or total production that I’ve actually been able to produce within a specific batch as well. Let’s go on. Obviously we’ve got some downtime isolated there, and I can also use that to then say, you know, what? What was my actual duration of these cycles? And I can also go and say, well, my mes or manual capture downtime, I can overlay that piece of information as well. So the more context we add to it, the more KPIs and more questions we can actually start answering about the information or the data.
07:55
So by having my downtime information, I can now know what was my downtime cause what was the duration of my downtime, what is the frequency of that downtime, as well as any potential loss in production or loss in energy while I was in that downtime state. All right, so where are we now? We are currently at the end of the shift or the end of the day or the end of the production. What was my shift today total of energy usage? What is my total for the entire day or for the process? Well, we can also do the kind of roll ups of that. So I can tell you what is my current hourly shift production or what is my day to date currently value.
08:39
And I can also then look at the previous hour to grow and create KPIs to tell me what is my rate of change based on the previous result or the previous time bucket that I’ve aggregated the data from. We can slice this data now by our shift context, all right? And we can now start to add shiftly KPI values as well. So what is my total shiftly production? And what if I want to know? I’m halfway through the shift. I’m not done where potentially I’m going to end up with. All right, so again, I can use the historical context, and I can go and create a very simple run rate. So what is the run rate of my past 4 hours? And based on that run rate, where can I suspect to end up at the end of the day?
09:31
Now, we have the capability to project data within flow. So I can take that. You start that run rate. I can ask the flow engine to go and calculate that, and I can project it into future time. Oops. I can project that into future time buckets. Clark, I’m signing up for your clicker 101. So there we go. I can go and project that and see potentially where I’m going to end up at the end of the day. Now get your data from your mes to say what is the actual plan or what was planned for the day. And now I’ve got a very actionable KPI to tell the operator. Listen, you either need to step up to produce more because you’re not going to hint that end of the day target.
10:15
All right, so based on two points, yes, we added context from a lot of different other data sources, but two points was able to generate a whole bunch of information and KPIs just on those two points that we generated from our production environment. Okay? And this is what I do. This is what I love. I love taking data, turning it into actional pieces of information to then at the end of the day give you an actionable KPI would say, are you going to meet your targets? Yes or no? All right. What type of analytics have we been doing for the past? I don’t know, 1020 years, 40 years is maybe a little bit much, but we’ve done what we call descriptive and diagnostic analytics. Always after the fact. Right? It’s what happened in the past hour, what happened in the previous shift.
11:10
You’ve got your typical kind of PDF, shiftly report that was posted out, and then you’re going to try and say, okay, I understand, I didn’t make my shiftly target. What happened? What is the additional context that I can add to that data to provide me with that diagnostic type of analytics? Now, there’s nothing wrong with this. We’re going to use this today. We’re going to use it probably forever, but where are we going? What is the next two big things that people want to do with their information? We talked about it this morning in the digital transformation kind of discussions, is everybody’s talking about machine learning, everybody is talking about AI. All right? So if you want to move from prescriptive and diagnostic analytics into this world of predictive and prescriptive analytics, what do you think we need to do to achieve that goal?
12:04
Well, the only way that we’re going to achieve that goal is that we need to have a place that I can send all of this nice, clean, contextualized data to, the same way that I do every day, and it’s in a repeatable way, because these type of analytics needs enormous amount of data to actually perform at their best possible way. Now, I can’t use old industry 3.0 kind of methodology to achieve this. And why can’t we do that? Well, it’s almost like the typical saying, putting lipstick on a pig in Afrikaans. We’ve got a different saying. We say, aldra rung a Blaine of sets of Lekadung. But we cannot take an industry three approach to analytics. All right? We’re doing it for the real time world. We’re talking about UnS concepts. We’re talking about embracing the digital transformation on that level.
13:03
So why are we not doing it on analytics perspective? All right, so how did we do analytics in the industry 3.0 way? What have we done? Well, we’ve got an SME subject matter expert. He came to you and he said, well, I need to achieve a specific analytics goal. We went as integrators and we built him that. All right. It was driven by a business course. That’s great. We then identified a typical type of application to do the work in. Nine times out of ten. What was that application? It was probably excel. All right, so we created this nice application, all right? And the steps that went to to create that application was, well, obviously we had to connect to some data, we had to standardize and cleanse that data. We added the context that necessary for the guy to get the answer.
13:58
We performed some calculations and we validated the result. That sounds pretty much what I said right in the beginning, right. And that’s the steps that we go through to create nice, actionable KPIs to solve some other business value or discover value within our data. The problem is that the more went up the pipe to actually get to that point was a lot of time and effort and money involved. And the problem is it was isolated to that app that was selected right in the beginning, either in excel or whatever the technology was at that point in time that was selected. How do we scale this? How do we take that excel document and we scale it to business case number two? Business case number three, business case number four?
14:48
Well, it wasn’t really possible because what ended up happening is we just created another one for application number two or business case number two. We created another one for business case number three, et cetera. So we ended up with, I don’t know about you guys, but I’ve seen Excel worksheets. If you open it up, it takes 2 hours to load because all the worksheets and the stuff at the back end just to get to all the data points that it needed to do the task. So we redoing a lot of stuff. We redoing connections, we redoing KPIs, we redoing calculations, and how do we share that to the actual people that needs to use them? So what we need to do is if we want to scale, we cannot create these Stowe pipe architectures anymore.
15:35
It’s not going to allow us to scale in the analytics environment. So one thing we need to create is what we call a single source of the truth. We need one place that we can connect our data silos together with and have one place to do all of that integration and KPI work. All right? Else we’re not going to scale. And we need collaboration with all the subject matter experts in your organization to get that working. At the end of the day, we need to make sure that we apply the correct data governance. Right? What do I mean by data governance? If I’ve got a KPI that is required by application number one or application or use case number one, two or three.
16:21
How do I make sure that the KPI or the calculation that I use is exactly the same for all of those applications? And then very importantly, we need to be able to scale the solution. All right, this is where flow comes in. We will never ever be able to scale if we take an industry 3.0 approach to analytics, just like we’re not going to stale if we take an industry zero 3.0 application to our underlying scalar information world, right? So let’s look at an example. We see this a lot. If you walk into any meeting room on a plant, you’ll probably see these whiteboards, right? There’s a guy laughing. Great few problems here, all handwritten. So where’s the order trail of that? Where does that data come from? Pie charts. Ooh, I love them. Not great. The human eye cannot distinguish angles.
17:26
So if I have a pie chart that’s very similar to the one side to the other, the human eye cannot actually distinguish which one is bigger than the other. Right? But the biggest problem that I have with this kind of ecosystem is that it’s all individual pieces of equipment or pieces of information that’s being presented. How do we share and how do we make sure that some of the work that I’ve used to get this data is potentially work that I can share on offload to the app that’s being used in this piece of data. So there’s no way that I can go and take these and extend it to the entire enterprise or to share this information with anybody in the organization.
18:11
So what we need to do is we need to go and look between these different applications and we need to say, okay, you know, what is common between them? Oh, there might be an integration between these apps that’s very similar. There might be a KPI or a calculation that I did here that’s going to be required in the use case for all of these applications. Or I’ve got a very unique use case that I actually need to do some data cleansing only on this part here. But the point is, I need to have one single place that I create all of this infrastructure, that all of the applications can leverage that same result. Right? And that’s our mission.
18:50
Our mission at flow is to give you that one single hub, a place where I can connect to all of these stove pipes or silos, and have one place that I can share all of this information out to any other piece of software that might need to use it within the organization. We see it as a hub in the middle where flow becomes the analytics hub that connects, consumes, create the context and shares it out to all of the different application. All right. Now, what is the benefits of this? What is the benefits of having this analytics hub or the scale of analytics? Well, you can utilize any work that you’ve done for any of the other use cases from the previous ones. If I already connected to the canary historian in use case two, great.
19:39
That connection is live and running and I can utilize that in use case number two or three, it enforces data governance from that perspective, because each and every KPI and calculation is going to run exactly the same time with the exact same code, with one place, no more different excel versions of KPIs and calculations, as well as a proper change log of when values change. And what was the change to those values or calculations. It’s highly contextualized information and it’s immediately available. What flow does in the background is we run on a cyclic engine poll time where we will connect to these data sources and pull the data out periodically to give you the information available when you pull it. We never do things on the fly.
20:32
So when I open up a report or a dashboard, that’s not the time to connect to the source, get all the raw data, do the data cleansing, do the aggregation, do the KPI, and then present the value back. It’s already stored in our database, it’s already contextualized with all the other information that you need, and it gives you then that highly contextualized information that you can make your decisions on and very important. And I think this is probably the biggest thing for me. Sure, me and Yaku always talk about three letter acronyms, and here I go and blast three acronyms all over the screen. SME collaboration. So subject matter, expert collaboration, anybody in the organization must be able to get to that information. Anybody in the organization must be able to use that to make actionable decisions and to make their job easier.
21:20
All right? And yes, we do play well with our stack ignition canary, but we need to also have a little bit of agnostic from that. I need to have a little bit of analytics freedom because I need to be able to connect to, yes, I’m going to say it, and avivo historian as an example, all right, there’s multiple historians that run on our plant floors, and if I really want this to work, I need to be connect to all of those to provide the additional context to all of my information and create that analytics app. Freedom or interoperability between all the percible silos of information that I might have on the plant floor. All right, so I think we spoke a little bit about it. Rob, how do you choose? What do you need to do?
22:09
How does this look from a strategic or success cycle? It’s like, shift a success criteria perspective. Well, you need to know, who are you selling this to? All right, where are you going to add value? And we see that we can add value to pretty much the entire value chain within your organization, all the way from your plant managers and process engineers at the bottom, all the way up to your executive leadership and data scientists and analyts that use the data. If we look at plant managers and operations, for us, it’s all about creating champions within the business. How do we create a champion out of an operator or a plant manager? Well, we give him the information or the KPIs that he can use to drive each and every one of those shift meetings that it’s in.
23:08
We had a podcast and a session myself and Yaku around actionable information. And how do we use that? Quite an interesting topic to go listen to. But the whole point is that we need to start driving meetings and get to an outcome of what do we actually need to do after the meeting without having to fight about is the number 50 or 54 on the screen, right. And the only way we’re going to achieve that is if the guys trust the value that they see on the screen. And that is if you have one single source of truth of that value. And then obviously we’re never going to get away from the human factor, right? We always have to have a place where someone can type in a manual data point. The human is never going to go away.
23:59
So we still have as much as we’re going to automate and embrace digital transformation, there’s always going to be some context or some piece of information that a human is going to enter to us. And that’s also a very critical part for us, is to have that capability, to have the human provide the additional context to the data as well. All right, how does this look for creating a champion within the executive leadership? Well, they can sleep sound at night knowing that there is information governance that’s been deployed from a flow perspective. And the way that we do it is with our templatization technology. If you have a multi site organization, how do you do benchmarking between those sites? If you don’t know that each and every site is running the exact same version of a KPI? We enforce that with our templates.
24:50
And we can also standardize different time frames within a site, different sites, different days, different shifts, because we need one way to actually do that. Benchmarking between a time period perspective as well. All right, for the number crunching guys, the data scientists and analysts, well, we need to give them a place that they don’t have to interrogate four or five different systems to get the data that they require. Now, they need to get data from an aviva. Now they need to get data from a canary at one site. They might need to get data to all four and five or six of them. How does that look? How do you massage the data when you get it from those systems into a way that they can actually run it through the same kind of model?
25:39
Now, what we’ve done is with, that’s a four letter acronym, SSOT. Single source of truth means that I can interrogate data from, and it can be raw data or calculated data from these different sources, and they look exactly the same. We’ve got a standardized way that we present that data back to these guys, that they don’t have to do the actual mapping of that data. And that’s exactly what we try to explain here. We’re combining these multitude of tags and data sources into one model that everybody can access and they don’t have to care from which historian they’re actually getting the data from. Now, this has been used by quite a lot of companies. So we help companies in the world to mature from a data maturity perspective.
26:29
And these are just some of the companies that we’ve worked with from a global perspective, that we embrace this kind of multi site environment to help them to drive their digital transformation journeys. Cool. And with that, I already asked my questions. I already gave out all my socks. So I think it’s time for lunch. Thanks, everybody.