In this first episode of 2022, we speak with Carolina Torres from Cognite, an Industrial software business that helps transform industrial data into customer value.
SPEAKERS
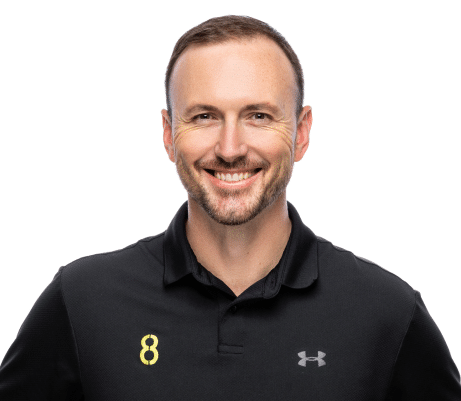
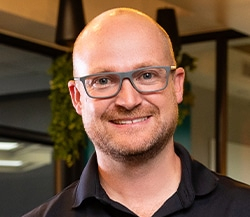
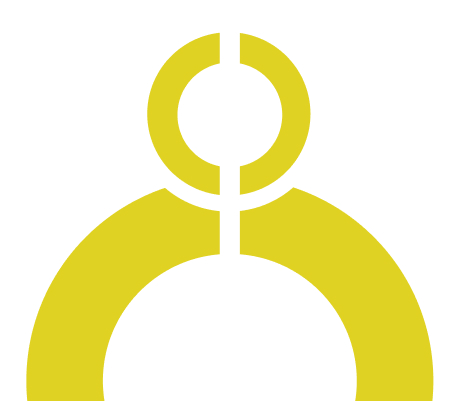
Transcript
00:08
Speaker 1
Hello, my name is Yaku and this is the Human and Machine podcast. To our first time listeners. Welcome and welcome everyone to a new year. Although it’s already February. Lenny.
00:17
Speaker 2
Yeah, it feels like we slept a little bit on this one. Yakuz. And it’s only in the month of February.
00:22
Speaker 1
I know it’s been Midland this year, but it’s all good progress. So of course, on the unmission podcast we aim to help you make sense of the latest industrial technology and the challenges, opportunities impacting manufacturing, production and sustainability today through some of the conversations we have with some amazing people. And we’re grateful to our guests that we’ve had in our short while that we’ve had the podcast that have shared their thoughts, insights, and often predictions, which is usually the exciting sizing piece. So you’re listening to episode 28, and if you’ve missed any episodes last year, make sure to catch up on our insightful conversations with Arlen Nipper, Walker Reynolds, Travis Cox from inductive automation. We had Vanesh Maharaj from PwC, Chris Clark from ABMBEF, and just many more good people. Lenny, let’s say hello. Lenny is of course my co host or host.
01:18
Speaker 1
I’m not sure what our relationship on the show is, Lenny, but it’s been an amazing one that I’m grateful for. But yeah, Lenny is with me today and we’ll get into the topic in a minute. But yeah, we’re here to share, get context, help, and most importantly, learn from each other. And it feels like all things our community needs at the minute. So Lenny, our leading training topic last year was without a doubt, data.
01:45
Speaker 2
Yeah, I think the problem isn’t anymore storing data or getting access to data. I think the problem these days is what do you do with the data? Turning that data into insight, I feel, is the biggest problem, the biggest challenge and biggest opportunity that we currently all about adding context to that data. At the end of the day, we want to empower and we want to make actionable decisions based on that data or that transformation of data into information. And that’s where, boy, I work. And that’s my little bit of a passion about that transformation, to make actionable decisions.
02:21
Speaker 1
That’s right. It’s not just about the data, it’s about data to value. And I think it was Rowan from AbMbev who said not all data is valuable nor created equally. Yeah, and while most folks are trying to solve production, corporate and energy and sustainability challenges, the data to value journey seems to be the most complex. So we heard about last year. We heard about MQTT, the unified namespace open architectures. We also learned about some common traps, the analytics chasm data swamps technology tail chase, and of course the problems which were plentiful, scalability, technical dates, listing context.
02:59
Speaker 2
A new term, data ops has been born.
03:02
Speaker 1
Data ops. That’s right.
03:04
Speaker 2
So yeah, making sense of what that is and what benefits a data ops platform can actually introduce into your organizer.
03:11
Speaker 1
I think we’ll continue with that trend because it’s definitely not that theme. Sorry, it’s definitely not a theme that we’ve done exploring and delving into. So the question is really, how do we deploy strategies to collect and contextualize data, very importantly, democratize it and share it as decision making information, and especially with the benefits and advances of AI and machine learning. So, yeah, to guide us through some of these talking points today we’re excited and grateful to host Carolina Torres, who is the executive director of energy industry transformation at Cognite. Carolina, welcome and thank you for joining our Humble podcast here in South Africa.
03:49
Speaker 3
Thanks for having me.
03:51
Speaker 1
We’re looking forward to this chat. That was probably a long intro, but those are just some of the real conversations we’ve had on the topic over the last little while.
03:59
Speaker 3
I’m looking forward to talking about it.
04:02
Speaker 1
So Carolina, do you maybe want to give us an idea? I would imagine some of our audience have not heard of your business to maybe give us a bit of context about your background. First and foremost, we did have a quick chat about that before we started the podcast, and it’s certainly an unconventional, interesting background.
04:22
Speaker 3
Sure. So I’ve spent about 30 years, 30 year career in upstream oil and gas, mainly around subsurface and wells. My background is in geology, but I spent quite a bit of time on figuring out how to develop oil and gas fields, drill wells into them. I’ve also worked in major projects and finance, but the last five years of my career I led BP’s digital transformation for subsurface and wells, and I also worked on the energy transition. BP was very focused on reimagining energy and then also reinventing themselves as an energy company as opposed to just an oil and gas company. And what does that transition mean and how do we remain profitable while we do it? That was a topic of a lot of conversations and a lot of strategy work.
05:13
Speaker 3
What I learned from my 30 year career, plus the five years focused on digital transformation and change management and strategy, is that transition is a data problem and basically everything that digital transformation is a data problem. And we have started out, and I’m guilty of this myself in my career, starting out thinking that it’s all about the tools and the applications, but it’s not. It’s really about the data. So when I decided to leave BP, I wanted to work for a company that focused on that. That focused on how do we do data differently to enable digitalization, process automation, and ultimately to change from the old hydrocarbon based energy world that we live in to something that’s a little bit more sustainable and better.
06:04
Speaker 3
And I joined Cognite about a year ago, really wanting to solve this data problem as a foundation to transforming the way that we deliver and use energy in the world.
06:20
Speaker 1
I love it when people are passionate about data. I love this conversation because I think when we speak about digital transformation, I think the common elements are well known and well understood, the technology, the people, the process. But I think very often, if you want to do anything that’s scalable, you quickly realize that the data is where that’s the hot source.
06:47
Speaker 3
Just going to say. My first year, the big focus was on developing toolkit. Oh, let’s make applications, or let’s develop these data science solutions. Let’s do a pilot to see if we can automate this or if we can do a predictive model here. And we spent a lot of time massaging, cleaning the data and building data models. And then we would solve a problem for one pump or one issue or one. Well, and then it wasn’t scalable or repeatable, and we’d have to do the same thing over again for the next. Well, or the next question that we had. And I think that’s where many companies have gotten frustrated, is that this sort of piloting. It’s PoC purgatory, is what we call it at cognizant.
07:38
Speaker 1
Oh, yes. It’s actually a graveyard.
07:40
Speaker 2
Yes, it’s one of my pet. It’s a never ending. The POC runs for indefinite. It never gets to a point. It never actually proves what it’s supposed to prove. I always have the saying, if a POC runs more than two weeks, it’s a project, it’s not a POC.
07:58
Speaker 1
And you would probably, Karanita, you probably would have seen this. A number of rfps, qs, T’s, whatever the document is, the number of those that we’ve seen, and probably you have as well, that does not have a defined business objective, which is just absolutely mindless.
08:16
Speaker 3
See cognite as being at the epicenter of three global megatrends, if you will. There’s the industrial digitalization, which was kind of old news. We’ve been talking about that for a long time, the energy transition, which is kind of the new buzword, and then workforce transformation. So those three things all require a very different mindset and a very different way of dealing and handling data. Industrial digitalization is about using data, and really, more and more data is becoming available as more and more industrial processes have sensors and are spewing out data like crazy. But it’s really about using that data for humans to make better decisions. It’s using AI and machine learning to help humans make better decisions.
09:11
Speaker 3
The energy transition is really about reducing the impact of our legacy energy systems while transitioning to something new, which is in the process of being invented and figured out. And it’s about reinventing the supply chain to turn it into a supply cycle, as opposed to a single, linear, one way trip to the dump. And it’s about an unprecedented level of collaboration and transparency across all the players. So if you are going to change from a supply chain to a supply cycle, that means you need enormous amount of transformation, being able to collaborate, and you need to be able to see each other’s data, from suppliers to operators, to consumers and regulators. You need that transparency in the data and the sharing of information in a way that we never have had to do before.
10:08
Speaker 1
I’m almost visualizing it as a flywheel, where we have exactly interconnecting points. But for that wheel to function as a wheel, those points need to be connected, understood, shared, and be transparent to the other. Otherwise, it won’t be a wheel, would it?
10:27
Speaker 3
Right. That’s absolutely right. And then the workforce transformation is really about transforming the work to be more data driven and empowering workers out in the field with all the info that they need in order to operate more efficiently or to operate with a reduced greenhouse gas emissions or wastewater, they need to be able to make on the fly real time decisions. And it’s about reducing the risk and exposure to danger, using robots and drones and things like that. But then again, more data that needs to be incorporated and brought in and contextualized and mixed with other information in order to inform a decision. So all of these things require a level of data operations that doesn’t currently exist. Generally, you can’t do any of these things using 19th century data management mentality and processes.
11:24
Speaker 3
Data management is about how you store the data, how you categorize and catalog that data, so that then you can pull it sort of in a one off way and deliver it. To me, the big difference between data management and data operations is a living, breathing, changing, continuously evolving thing that gives you unlimited access. And so that’s kind of how I see those two things as being quite different. People are trying to still do data management with this tidal wave tsunami of data that’s coming at them from sensors and things in their industrial world and it’s not going to work.
12:10
Speaker 1
Yeah, with the advent of cheap networks and devices, we do have this deluge of data just seemingly too much for people to understand what to do with, store it efficiently, contextualize it effectively and share it out as useful information. That’s the gist of the challenge.
12:29
Speaker 2
And we’re still seeing that data is being kept hostage in solutions, in proprietary pieces of equipment.
12:36
Speaker 1
We call them black boxes.
12:43
Speaker 2
I had a quick look at the values of cocknut on the website and I think the one that’s very passionate for me and one thing that I feel very keen about is that data must be open to create value. I think if we’re not going to get that right and share that data and give the people the data that they need to make their decisions on, well, what’s really the point? So I love that value call of cocknite around that data must be open to create value.
13:13
Speaker 1
Yeah, for sure.
13:14
Speaker 3
It’s absolutely essential. And like Jaco was saying in the flywheel, if you think about the supply flywheel, I love know it all has to be open. And it’s not just about being open vendor operator open relationship, but it’s around the supply suppliers and the consumers even, and the degree of transparency that companies are going to need to have with regard to their sustainability information, both for the financial sector and for their consumers, that’s got to be more open too.
13:50
Speaker 1
So we mentioned industrial data ops. Maybe it feels like we’re only teeing it off now. Maybe as a departure point then industrial data ops, you would probably aware there’s a couple of solutions products on the market now being positioned and messaged as industrial data ops solution. Maybe you could share in your mind and your views what is industrial data ops, what it isn’t and what it aims to solve for in this data journey.
14:33
Speaker 3
Okay, maybe I can use analogy. I don’t know if this will work or not, but let me think about this. So if you think about in the olden days, everyone had a well in their backyard and whenever they needed water they’d have to get a bucket and walk out to the well and draw some water and pull it up and bring it in and do whatever they were going to do with that water right now, when plumbing was invented, the whole mindset had to change completely, because now you had plumbing, you had basically water on demand in your house. And that led to the creation of lots of multiple types of uses for that water. So showers and baths and a kitchen sink, and you could flush a toilet and you could have a hot tub.
15:27
Speaker 3
So having plumbing in your house is like having a data ops platform. A data ops platform enables you to create multiple different uses and slice and dice your data in multiple different ways. The data management world that I was mentioning earlier is basically every time you need data, you have to go to the well with your bucket and pull the water and carry it back. And for each different usage or question that you might have that you want to try to answer or decision that you might want to make, you have to go and draw the water with a bucket. Whereas with a data ops platform, it’s there, it’s available, and you can use it in any way you want. It’s at your fingertips. I don’t know if that, but it’s setting up a pipeline, right?
16:20
Speaker 3
You have multiple sources of data in an industrial world. I mean, I’m most familiar with oil and gas, but you have Osipie data. You have data from SAP, that’s financial data. You may have data that’s in subsurface data, that’s in slumberger product. You mentioned all these different silos. So every different little thing that you do has a little pool of data that’s kind of locked into a little silo that has proprietary data model. And you have to bring all of those different sources together. And if you don’t have a data ops platform, if you’re just data managing, somebody, a human or a team of humans, has to take that data, bring it together into one place, and map out the relationships and link up the different pieces of that data.
17:07
Speaker 3
And then the other thing that you all mentioned was about curating, because not all that data is necessary, maybe only parts of it. So you have to curate the right bits, you have to clean it and then create a data model, which you then can do your query or make your decision based on. And that is the bucket story. Whereas if you have a data ops platform, you have automatic pipelines to all of these data sources, and you’ve got machine learning and AI algorithms that will do that, bringing the data in and creating those relationships across all the data, curating it for you, contextualizing it, and then creating an API or an SDK or some kind of a faucet that lets you access it. That’s what a data platform does.
17:57
Speaker 1
Well, the latter part of what you’ve just said is super important. I think having the ability to collect from various disparate types of data sources, contextualizing, curating, whatever you need to do to clean and aggregate and make that data relevant is likely. I don’t want to say useless, but you negate the value of that if you don’t have the ability to share it with its context to other business applications, sources, people, and different roles within organization, and the format and context that those people and those systems needed it.
18:37
Speaker 3
That’s absolutely right. That’s what we call our API SDK layer is basically all of the faucets that are out there that anyone can hook to. And that’s where the principle of openness comes in, because we’re not just, I mean, we do have some applications at cognite that we can sell to people, but you don’t have to. You can get a third party, or you can write your own citizen developer code or whatever it is that you want to do with that data, and it’s open, anyone can use it.
19:11
Speaker 2
I always use the analogy of a time machine, and the reason I say a time machine is I think if you don’t have that faucet having this immediate tap, that you can just open up and have this information almost in real time available. We always seem to do things reactive. You go, as you said, you go spend so much time collecting, massaging, cleaning, that the data to make the decision on is almost old again. It’s a reactive after the shift, after the day, after this process order has been run. It’s a very reactive way versus a more real time and preactive way of actually having data that you can influence way production is going, not reactive actually, while it’s still busy happening for that shift or for that day.
20:02
Speaker 2
So it saves not only time, your personal time, that you actually do the job that you’re supposed to do by having information available also saves the time of your company, reducing cycled counts, et cetera. So it’s got massive value having an automated data stream available for you that is available on tap. Almost. We can coin it that on tap.
20:26
Speaker 1
Let’s call it value on tap.
20:27
Speaker 2
Value on tap.
20:29
Speaker 3
I think one other element too that’s quite interesting is that a lot of times the data silos, where the data sources are, where the data resides, we talked about these proprietary vendor applications and where the data sits in there. Oftentimes those are license based systems and they’re very discipline specific. So the geologists all have licenses to patrel in Slumberger Patrell, which is a software that helps you to make maps and things. The engineers have licenses to some other software where all the engineering drilling data is housed, and the finance folks have licenses to the SAP data, where all of the purchasing and logistics sit. And it’s all very isolated and not very democratic. In other words, geologists don’t have access to drilling data or financial data. Financial people don’t have access to drilling data or geology data.
21:33
Speaker 3
And so that actually stymies innovation quite a lot, because oftentimes if you bring all the data in and contextualize it, you also democratize it. It’s not just that it’s open to anyone, but multiple people who think in multiple different ways, have access to the big picture and can maybe see things that people in isolation, in their discipline bubble, with their software bubble and their data bubble, wouldn’t be able to see.
22:03
Speaker 1
Yeah, for sure. I think the automated piece of what you just said, plus a team of people to curate, plus the experts, I’m going to call them experts loosely. I think experts is probably today, in our world, experts is. I think experts are nonexistent. Or at least that’s when you lose your expertise, when you call yourself an expert. But having the dependence and the reliance on all of those resources, tools, applications, the more of that you have, I think that’s where the technical debt comes in. And you mentioned earlier how all of that inhibits innovation and the technical debt is such a big aspect of that, isn’t it?
22:47
Speaker 3
Yeah. Domain knowledge workers is an alternative to experts, but it talks about the different knowledge domains that people have. And again, I just think it’s super important for people to be able to have all the information, even it might be outside of your domain, but it might actually impact your domain. So it’s really useful to have that democratization of data.
23:17
Speaker 1
Absolutely.
23:19
Speaker 2
I think it’s also to break habits. I mean, if you don’t show people the. I’m just going to use financial data as an example. If you don’t show people financial data in context to time series process data coming from a fraud and the actual actions that an operator takes having potential, not only financial implications. We’re probably going to talk a little bit about energy and how do we drive effective energy usage. But if you’re not going to show the actual effect of what he’s doing on the plant floor based on financial or energy KPIs, we’re not going to break habits. We’re not going to make that guy think about what he’s doing, because again, we’re giving him the silo in isolation just to operate the plant or whatever. The case is that he needs the context. There’s no context. Yeah.
24:13
Speaker 2
So I think that’s critical. And as you said, it’s not playing open cards here. Right. Here’s all my financial statements of the business. But having that context around just a little bit more, if you now change this set point or stop this device or enable this new pump, how does it actually infect, not only financially, but also from an energy perspective, how does that impact?
24:38
Speaker 3
Yeah, it’s really critical now. I mean, we have some customers in Canada, and Canada has just recently instituted a carbon pricing system. And it’s kind of in a tranche system, so that if you exceed a certain threshold, then your pricing, like, say, doubles, and then if you exceed that, then it’s kind of an exponential curve. And so you have people out there whose job it is to improve or optimize on production only, and they’re only looking at their production numbers. But it actually comes to a point where if you produce above a certain threshold, you’re actually losing money. So you need to be able to see your greenhouse gas emissions impact for every decision that you make, and you need to be able to do it real time.
25:29
Speaker 3
It’s not good enough to just go back over last year and look at your SAP receipts and say, how much fuel did we buy? And we’ll turn that into greenhouse gas. Convert that with a conversion factor into emissions. That’s way too late. That’s not an actionable piece of information anymore. You have to actually have it real time.
25:50
Speaker 1
Yeah, I wonder how the truckers feel about that.
25:57
Speaker 3
Mean, we’ve had some really interesting results from. There was one project that we did where were helping a facility optimize their meg usage. Meg is a chemical, ethylene glycol, which we use to prevent hydrate formation in pipelines. And it’s not a nice chemical at all. We really want to try to use it as little as possible. The problem is, if you don’t have real visibility to exactly what’s happening, you end up doing a sort of a scheduled utility of this chemical, as opposed to really only doing it when you need it. People err on the side of caution and overdose this chemical a lot because the flip side, if you get it wrong and you freeze up your pipe, you have to shut down your entire production. And it’s very costly.
26:56
Speaker 3
When we instituted this across six different assets, in one of our customers and the operators had the visibility of this, they started to really query and understand. They were able to see not just their meg situation, but also all of the facility’s meg situations. And so they were able to compare, understand best practice. Why does that twelve hour tower use less Meg than that other twelve hour tower? Why does that facility, which has the exact same equipment and piping design, use less than that one? And it was all about how we practice and what is best practice, what is the technical limit? And really having this visibility and this transparency around the data, the operators themselves started to compete with each other and say, well, how low can we go? How can we do this better?
27:55
Speaker 1
And when your operators are in that frame of mind and that drive and that’s the way they operate, then you’ve done something correct. And that’s the winning recipe. When the people that are at the coalface that probably have the most impact on the overall picture, when they have that sense of awareness and visibility to what their actions cause and can contribute, that’s where you want to land with that.
28:19
Speaker 2
I think very important to that point is that if you have, in this case it was six facilities, if you have maybe 20, 30, 40, also very important to understand that you should have a thing that can scale that the benchmarking that you apply and the model or the template that you apply to that KPI should be the same for each and every one. So you can with confidence, actually make that benchmarking comparison. I think that’s a very important thing is that you should have confidence in the data making a decision. Nobody this day and age should sit in a meeting or in a discussion around why is the number x arguing.
28:58
Speaker 1
About the validity or the correctness.
29:02
Speaker 2
The point should be what is the action that I’m going to take around why the number is a certain value, not why is the value xyz? And I think that’s an important thing is that people need to start trusting their data. And if you have a solid platform that gives you that solution, then you should be a four away actually utilizing that efficiently and getting to the bits that matter. What is the actual improvement or action that you’re going to take on it?
29:34
Speaker 1
Carolina, you’re from your background is oil and gas. So ours is a little bit more food and beverage. But we see it when we’re talking about energy and the energy challenge and the energy drive. We see it with something very simple, like utilities for example, in a food and beverage manufacturing environment. The ability to understand, I don’t know water, electricity, air, whatever the energy.
30:01
Speaker 2
It’s actually quite interesting because in food and beverage, we also use chemicals to clean and sterilize the production line after it. We have a story where they were dumping the chemical into the wrong pipe on the wrong and actually went to waste. And it’s a chemical that you can actually reintroduce into the process and reuse it over and over again. So, yeah, it sounds very similar. And I think that’s the point, is that doesn’t matter if it’s oil and gas or food and Bev, it still stays a data challenge to actually achieve or to see what is the business goal that you want to achieve with that.
30:40
Speaker 1
Seemingly a little bit philosophical, but it’s actually very pragmatic and practical. I mean, if we’re talking about sustainable future and energy initiatives, it’s almost unthinkable how we can understand, to reach that end goal or destination without understanding where we are at the moment and what we need to do to change or to pivot in a certain direction. And that’s the secret of the data.
31:05
Speaker 3
Yeah, absolutely. I mean, most of our customers don’t have a view of what their baseline is, right? In the oil and gas sector, these companies have made these incredibly ambitious claims around net zero by 2030 or 2040 or 2050 or whatever. And not only do they not have any idea how they’re going to get there, but they also don’t even know where they are right now, like, where the starting point is. What is our actual greenhouse gas emissions right now, or our wastewater, or all the different things that we’re trying to measure, chemical usage, power usage, et cetera.
31:45
Speaker 1
But just establishing that baseline is a data problem already at.
31:52
Speaker 2
Normally, what we see is if we create the baseline normally, what will happen with the baseline is the variability in that usage will be quite high by just having insight around what that baseline is, and the variability around the usage immediately reduces the variability. You’re not doing anything fancy even with it. It’s just the fact that you make.
32:16
Speaker 1
All of a sudden visibility and visibility.
32:18
Speaker 2
On what that is. That alone can drive less wearability in the actual usage of b water, wastewater production, or whatever the case is.
32:27
Speaker 3
Yeah, I forgot who it was that said you can’t change what you don’t measure.
32:35
Speaker 2
Exactly.
32:35
Speaker 1
Yeah, we have a couple of analogies that we usually refer to as flying an aeroplane without various gauges or driving a car without a dashboard. I mean, it’s simple and it’s so obvious, I suppose. But when we think about large, complex initiatives it’s almost something that’s sort of forgotten because of all the noise around the tech and the approach and the strategy and the people and the resources. It’s such a fundamental thing I wanted to be far to. So Lenny loves speaking about AI and machine learning as a subset, whatever you want to categorize. So when we talk about, I don’t know, Karolina, is it emerging technologies? Is it.
33:22
Speaker 1
I don’t know what the correct terminology of all of these really smart and clever initiatives are that potentially hold so much promise, but is seemingly also, to your point earlier, not that easy for people to scale. What is your view around those technologies at the minute, and how do you feel about the introduction and the adoption and the scalability?
33:45
Speaker 3
You’re talking about artificial intelligence, machine learning, and they span multiple different things. I mean, even things like computer vision, for example, or natural language processing, extracting information from old documents or immersive technologies.
34:03
Speaker 1
I mean, there’s so many subsets.
34:05
Speaker 3
Yeah, it is a very broad thing, but I do think there’s some really positive aspects to that with regard to, I mean, something as simple as computer vision and being able to fly drones over. We have a project with some power and utility companies where we automatically detect vegetation encroachment. So we can do that with drones or with cameras that they have, basically looking at vegetation and using a machine algorithm to recognize when trees or other things are encroaching on power lines, which then reduces the risk that would be hundreds and hundreds of man hours of people driving out in trucks and measuring stuff that can be done very quickly and efficiently using satellite images or drones.
35:06
Speaker 2
Think about all the gasoline that.
35:10
Speaker 3
Exactly man hours and driving hundreds of truck hours and fuel for that. There’s a lot of things that technology is doing that I think is good and positive on the sustainability side of things and also removing danger people from dangerous got. We work with Boston Dynamics, and we have a spot the robot dog. We do a lot of programming of spot for some of our customers. And for example, we’ve got algorithms that we’ve written where we take data from an asset, a facility, and if there’s an alarm of a potential gas leak, for example, we can send spot in, and he’s got a sniffer on him where he can sniff out all kinds of different chemicals so that you don’t have to send a person in there.
36:10
Speaker 3
You can send a robot dog into these nooks and crannies within a facility to determine if there’s a danger or a leak or some other issue.
36:21
Speaker 1
That’s incredible. We have in the mining industry. So the mining industry is really the stronghold there in South Africa, and it has been for many years. But inevitably, any one of these mining sites that you go to, there’s a lot of tested and tribal knowledge that exists within the workforce in those industries.
36:40
Speaker 2
Right.
36:41
Speaker 1
And inevitably, at any one of these plants, there’s always usually a fairly senior person that’s been there for probably his or her entire career. Yeah, that’s where they started their career. And you start speaking about these kind of technologies, and they’ll quickly explain to you that they can simply walk through the plant and year if a turbine or something is not running efficiently, as it should. And that’s fantastic. So the first thing you do is congratulate them for their skill and their experience and their knowledge, but then explain, all right, and if you’re no longer at the site or the splant, who’s going to have the intuition or the skills and the knowledge to know that something isn’t running as it should just by keeping an ear open? I mean, you still find a lot of that kind of mentality as well.
37:32
Speaker 2
That was the coolest thing. When I saw what spot actually can.
37:36
Speaker 1
It’s phenomenal.
37:37
Speaker 2
It’s audio recognition on pieces of equipment. It’s such a simple. It’s actually a very simple idea to compare what is a normal audio wave for a piece of equipment versus when things go wrong.
37:50
Speaker 3
Yeah, well, and combining it with other data as well. Spot can read analog dials and recognize spills and all kinds of other things. And it’s great. You get image or you get an alarm or some kind of thing like that, but it comes into cognite data fusion and then gets combined with all of the other, the PNID, which is like the big blueprint of the facility as part of it. And then you automatically have access to the age of the equipment and what is its shelf life and is it ready to fail? Or there’s all kinds of other data that’s associated with that you can do analysis on and say, well, is this likely to leak now? Or it’s brand new, or maybe we did some maintenance on it and somebody forgot to shut something off or. You know what I mean?
38:43
Speaker 3
You have everything, all that data at your fingertips.
38:47
Speaker 1
Yeah, it’s very real. As examples, it’s absolutely very real. So we’ve spoken a little bit about the data challenge. We’ve spoken about the approach, the environment that you create through industrial data art. We’ve spoken a bit about emerging technologies and all of its subsets of artificial intelligence, of which there are many. I think once we understand the approaches, what needs to be done, let’s just call it as what’s next towards digital transformation. I think a lot of individuals and corporates, they view the journey as something that comes with a couple of hundred million price tag. It’s going to be done over 20 years. They’re not entirely sure necessarily where to start. They should start with a data foundation, but they’re not necessarily sure where to start, how to go about it. And it’s seen as one very long journey with very few milestones.
39:47
Speaker 1
We always talk about an agile approach, small intermittent wins, gains, prove some RoI, move on to the next one. Can you recommend any kind of investments, infrastructure, or just any recommendations to put in place to consider to help this transition towards not digital transformation specifically, but the end goal of energy and just a sustainable future?
40:19
Speaker 3
Yeah, I think that we need to move away from the thought that there’s going to be a piece of software or an application that’s going to solve the problem, that setting up a data foundation is actually good for all purposes, especially if you do it with an open system, that you’re not going to regret that investment. Because even if the software that you picked this year to help you make a given decision goes away or becomes not useful anymore, that data foundation is still there, and you can just plug in another software if something better comes along, or write your own or. A lot of people are investing in educating or upskilling their existing domain knowledge workers to be able to do data science and analytics. And so whatever data platform you invest in is going to be a good investment for all seasons.
41:23
Speaker 3
It’s going to be an investment that lasts.
41:26
Speaker 1
Yeah. You mentioned training and skilling up. Often we would talk about when Skynet became self aware, artificial intelligence and all of these sort of emerging tech. The immediate thought of many very labor intensive industries is the loss of jobs and workforce, where I think the view should rather be one of what skills and what learning do we need to deploy, equip that workforce with right now that will be needed once we do that, because that transition is inevitable. But instead of recognizing the loss, it’s perhaps recognizing the opportunity and sort of preparing for that now.
42:12
Speaker 3
Yeah, I was just reading this really funny article. Let me just acknowledge what you said, that resistance to change is very real and people, and there’s a lot of fear around what change will bring and how jobs will be impacted. And I think there’s a lot of kind of scare stories about how robots and drones are going to take over the world and everybody’s going to lose their job and things like that. My feeling about that is that, yes, there probably will be a loss of quite a lot of existing jobs, but that’s happened anytime throughout history. If you go back through the industrial revolution, or I was reading this really funny article called the big crapple, which was about New York City and how it was very dominated by horses, and they had so many horses.
43:03
Speaker 3
They had something like, I don’t know, 10,000 horses in New York City, and they were just kind of being buried under manure. And there was a whole industry around horses, right? There were stables, there were stable hands. There were people who did nothing but pick up horse poop. There was people who transported all the manure out to the farms that were around. I mean, there was an entire economy that was based on horses around the turn of the 20th century. And then by 1915, it was over. It was done. It was horseless carriages, it was cars. And so there was a whole bunch of new jobs that had to crop up as a result of that.
43:47
Speaker 3
There was people who mechanics, and there was chauffeurs, and there was a whole nother sort of industry that rose up, not to mention Ford’s factory and the invention of the automated, what do you call it? Assembly line. And I think that this is not any different. This is another sort of revolution in the way that we think and the way that data is managed and the decisions are made and the way that industry is going to work. But there’s going to be a lot of new businesses, new jobs, new actually, probably whole new sectors that are going to crop up as a result of it.
44:32
Speaker 1
When we look back in hindsight now, it wasn’t even a blimp. It was almost a natural progression, almost seamless. It just happened at that time. It was probably sort of like to where we are now, scary, intimidating, a lot of uncertainty. But again, looking back in hindsight, at least historically, there wasn’t even a blip. It just happened.
44:56
Speaker 2
It’s quite interesting because I take your well versus faucet example and the story about the horse and the car, because when Henry Ford introduced the mean, people said, no, that’s not what we need. We need faster horses. Give me a bigger bucket so I can get more horse out of the well. That’s what I need. But changing the mind. No, I don’t need a bigger bucket. I need a faucet. I need something that I can open up in the kitchen, open up in the bathroom, open up in the shower and give different answers, but it’s still coming from water. So yeah, that’s quite an interesting analogy.
45:44
Speaker 1
We’ve discussed wells, horses, spots, but I mean, Carolina. I mean, that’s why it’s so important to have these conversations and have it openly. It’s not the kind of innovation talk that happens within strategic teams anymore. We need to all have these conversations as a community and it’s important.
46:10
Speaker 2
And as a business, you always get the guy that’s going to say, I don’t need a KPI to tell me how good am I doing my job or why are you spying? Just that notion that information should not be held accountable as a stick. It should rather be something that’s going to save you time and energy and money in your daily job instead of spending hours on sites manually collecting data, looking at stuff in isolation to even try and figure out what went wrong.
46:44
Speaker 1
Definitely that’s also a cultural aspect.
46:46
Speaker 2
Right?
46:46
Speaker 1
Carolina? I think any change within a business, whether it’s around information strategy and data aggregation and information, there’s a big cultural drive within the business, and typically from top management down, that’s super critical. We’ve had a couple of examples in certain cases where once the information is exposed and shared, there’s a reluctance to hide it again because it exposes certain inefficiencies. It’s mindless to think that it happens, but it absolutely does. But that’s where the cultural aspects of that drive within the business and the leadership is so important.
47:24
Speaker 3
Yeah, I mean, change management is a whole other podcast, right? I mean, it’s very difficult. It’s very difficult human management. In my experience, people that are on the front lines, that are making. That are really struggling to make the right decision and get their hands on the information that they need in order to really make a good decision, are very positive and eager to do things in a different way. And executives are, because they know that it’s going to gain them efficiency and reduce costs and accelerate production and reduce footprint, where you really run into this sort of middle layer, which we used to call the gumbo layer at BP, which is sort of director level.
48:21
Speaker 3
People who have my age, I guess, or my level of experience that are directors or vps that have invested 30 years of their career doing something in a certain way, and they actually have sort of restricted elite access to information, which then gives them power within the company. And when you democratize the data and you make it all transparent and you do things in a really different way, that’s very threatening. And that’s something that I think a lot of companies run into is how do you engage that middle layer and make them feel like this is something for them that’s actually beneficial for them?
49:08
Speaker 1
It almost makes a couple of those folks in those roles sometimes irrelevant. It’s an entire role that exists that’s potentially replaced with a very smart report.
49:24
Speaker 3
They might see that, but actually you still need those folks to lead and to have vision and to help frame and make decisions. It’s important, but maybe you don’t need as many of them. You’re right about that. And maybe their role is different. It’s more of a thought leader as opposed to, hey, I have been a petrophysicist for 30 years and I have all of the global petrophysics data in this company. And if you want to know anything about petrophysics, you have to come to me. That’s no longer going to be a thing.
50:02
Speaker 2
Yeah, I think that’s also one of the big misconceptions about AI and machine learning is the notion that it’s going to do it’s going to make the change and it’s going to fix everything. It’s only going to give you the recommendation. A human still going to make the actual decision on what to do next. And I think that’s a bit of a misconception, especially when we talk about the new technologies. From that aspect, it’s just a recommendation on what needs to be done. You as a human make that final choice.
50:37
Speaker 3
Yeah, absolutely. And actually we talked about curation and how important that is. And even the contextualization, the mapping of the relationships across multiple different data types from different data sources. A machine can only do so much, but you actually need a knowledge worker, an expert, somebody who really understands the domain to continuously help that machine algorithm do that curation and that relationship mapping. It’s not going to be able to do it by itself. It’ll make suggestions. It’ll say, hey, I think that this well is located at this XYZ location based on the fact that I’ve seen it in these five other sources. Is that correct? Like somebody still needs know make that decision.
51:27
Speaker 1
Yeah. And innovate. I think human beings are we still the true innovators? And I can’t see that changing. Carolina, thank you so much. I’ve just realized we’ve been speaking for a while. Thank you so much for your time, your insights, that was really insightful. I enjoyed it a little bit philosophical. We always like that. Love the analogies. Any closing thoughts? And I’m sure we can share your contact information at Cognite with our listeners.
51:57
Speaker 3
Absolutely. Sure. No, I just think that what I would want people to walk away from this conversation is to think about their mindset and are they still kind of stuck in a little bit of a data management mindset as opposed to a data operations mindset? And you really don’t need to make a trip to the well with a bucket every single time you have a use of data.
52:22
Speaker 1
So that’s it, definitely. Data management versus data ops. Democratizing the think.
52:31
Speaker 2
I think for my last final remark, Yaku, I think we’ve said it so many times at so many different podcasts, but just make sure, again, use open standards, open protocols. Swapping out from x to Z shouldn’t matter. As Carolina also mentioned, make sure whatever you deploy, make sure it’s just based on open technologies. Sorry. You know, it’s one of my pet peeves.
52:57
Speaker 3
That’s great.
52:58
Speaker 1
So that was Carolina Torres, the executive director at energy industry transformation at Cognite. If you want to get in touch with Carolina, continue the conversation. Very insightful for us. Please do. And as always, send us any suggestions, comments, future topics to talk through. That’s why we here have great conversations, insightful conversations, and let us know if there’s anything that we’re missing that’s more relevant than some of the topics we’re talking about right now.
53:25
Speaker 2
From my side, second best, last, I promise. But the fact that data, the new notion of data being a commodity is just being amplified in every podcast that me and Yaku pretty much does. So please, if there’s any topics on data challenges that you guys want us to address, please let us know. But it definitely seems to be the topic of discussion. Definitely.
53:50
Speaker 1
So far, the series, fantastic. If you’re still with us, well done. It means that you either have a lot of endurance or if you really enjoyed the episode, which we did, it was lovely chatting with Carolina and just getting a lot of validation on some of these things we’re all passionate about. So that was episode 28. We will see you in a couple of weeks time for episode 29. We’ve got a couple of nice topics lined up, but, yeah, please get in contact at podcast at element eight, Sierra Z if you want to suggest any other further topics and comments. Lenny, thank you very much.
54:24
Speaker 2
Cool. Cheers, everybody.
54:25
Speaker 1
Cool. Thanks, everyone. Bye.